Unveiling the magic of generative AI
Unveil the power behind AI that crafts poems, designs images, and even simulates human banter. Generative AI is reshaping creativity. Dive into the tech that's redefining art and communication.
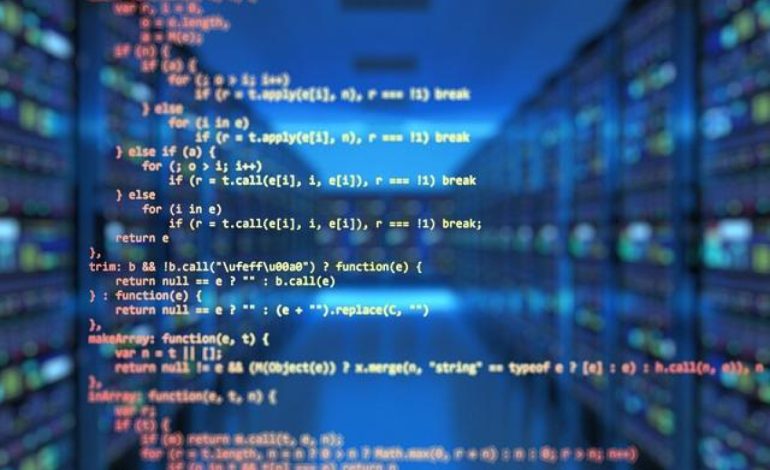
Understanding the future of creative technology: Exploring the transformative world of generative AI
At the forefront of artificial intelligence innovation lies generative AI, a captivating technology that’s the driving force behind sophisticated chatbots such as ChatGPT, Ernie, LLaMA, Claude, and Command. This technology also breathes life into advanced image generators like DALL-E 3, Stable Diffusion, Adobe Firefly, and Midjourney. Generative AI represents a segment of AI that empowers machines to assimilate patterns from extensive datasets and subsequently create original content reflective of those patterns. Despite its relative novelty, we’ve already witnessed a plethora of models capable of crafting text, images, videos, and audio.
The rise of versatile foundation models
Foundation models have undergone extensive training on diverse datasets, equipping them with the proficiency to tackle a broad spectrum of tasks. Take a large language model, for instance—it can effortlessly churn out essays, computer code, recipes, protein structures, and even humor. It can dispense medical diagnostic advice and, in theory, could even produce instructions for constructing explosives or biological weapons, although built-in safeguards aim to thwart such misuse.
Understanding AI and its subsets
Artificial intelligence encompasses a broad array of computational methods designed to emulate human cognitive abilities. Machine learning, a subset of AI, concentrates on algorithms that allow systems to learn from data and enhance their capabilities. Before the advent of generative AI, most machine learning models were trained to execute tasks like classification or prediction. Generative AI, however, is a specialized branch of machine learning that focuses on the creation of new content, venturing into the domain of creativity.
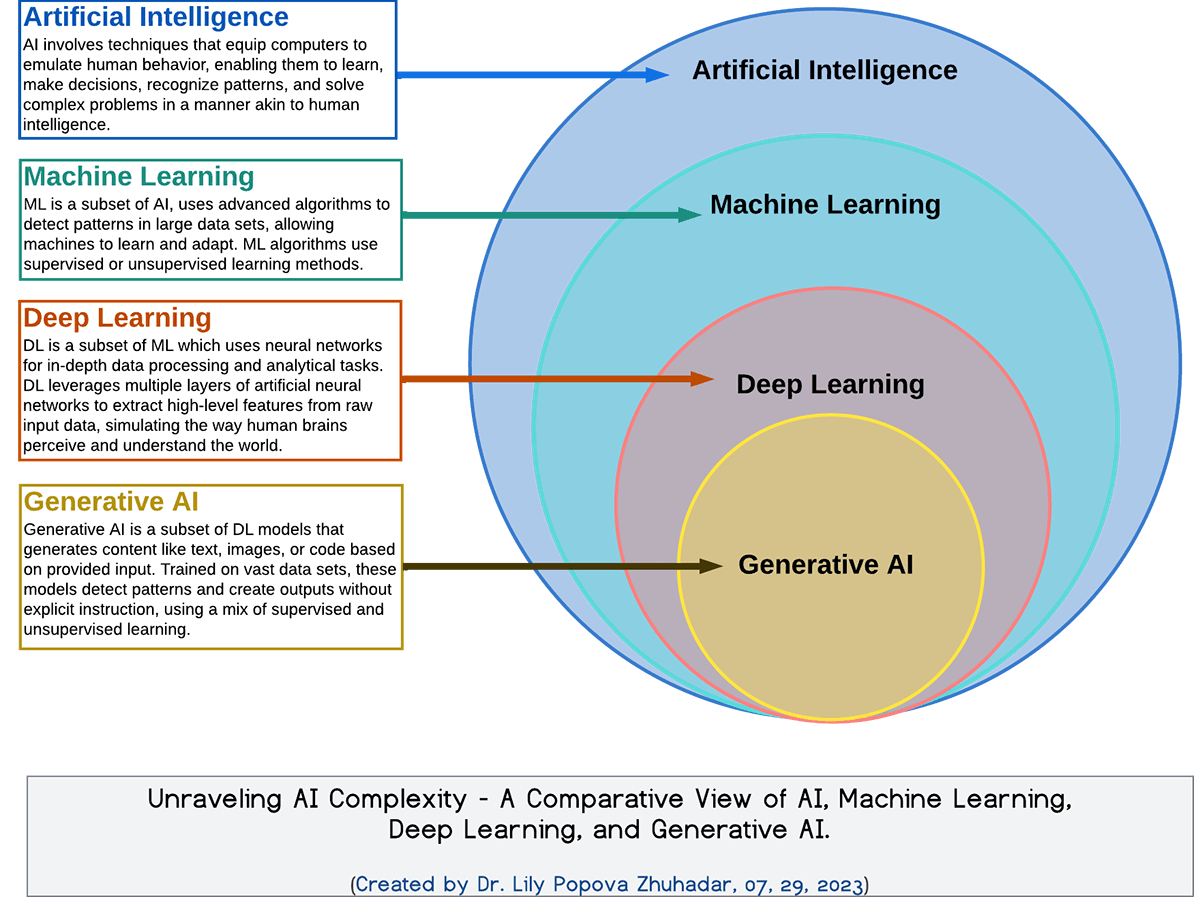
The architecture behind generative AI models
Generative AI models are constructed using various neural network architectures, which define their organization and the flow of information. Notable architectures include variational autoencoders (VAEs), generative adversarial networks (GANs), and transformers. The transformer architecture, introduced in a groundbreaking 2017 paper by Google, is the foundation for the current crop of large language models. However, transformers are not as well-suited for other generative AI applications, such as image and audio generation.
Autoencoders and their applications
Autoencoders operate on an encoder-decoder framework, where the encoder condenses input data into a more compact, essential representation in the latent space. The decoder then reconstructs the original data from this compressed form. Once trained, autoencoders can generate outputs from new inputs. These models are prevalent in image generation and have also been instrumental in drug discovery, aiding in the creation of new molecules with desired characteristics.
The adversarial dance of GANs
Generative adversarial networks involve a generator and a discriminator that act as adversaries. The generator aims to produce realistic data, while the discriminator’s goal is to differentiate between the generated outputs and actual data. This adversarial dynamic refines both components, leading to the production of content that increasingly resembles authenticity. GANs are notorious for their role in creating deepfakes but also serve benign purposes in image generation and other applications.
The supremacy of transformer models
Transformers are distinguished by their attention mechanism, which allows the model to concentrate on different segments of an input sequence to make predictions. This architecture enables parallel processing of sequence elements, which accelerates training. With the addition of vast text datasets, transformers have given rise to the impressive chatbots we see today.
Decoding the workings of large language models
Transformer-based large language models (LLMs) are trained on extensive text datasets. They utilize an attention mechanism to discern patterns and relationships between words, learning by predicting the next word in a sentence and adjusting based on feedback. This process involves creating vectors for each word, capturing its semantic meanings and relationships with other words. With over a trillion parameters rumored in models like GPT-4, these LLMs can grasp language nuances and generate coherent, contextually relevant text.
The phenomenon of LLM hallucinations
LLMs are sometimes said to “hallucinate,” producing convincing yet inaccurate or nonsensical text. This issue arises from training on data from the internet, which is not always factually correct. The models generate text based on patterns they’ve observed, which can lead to plausible but unfounded content.
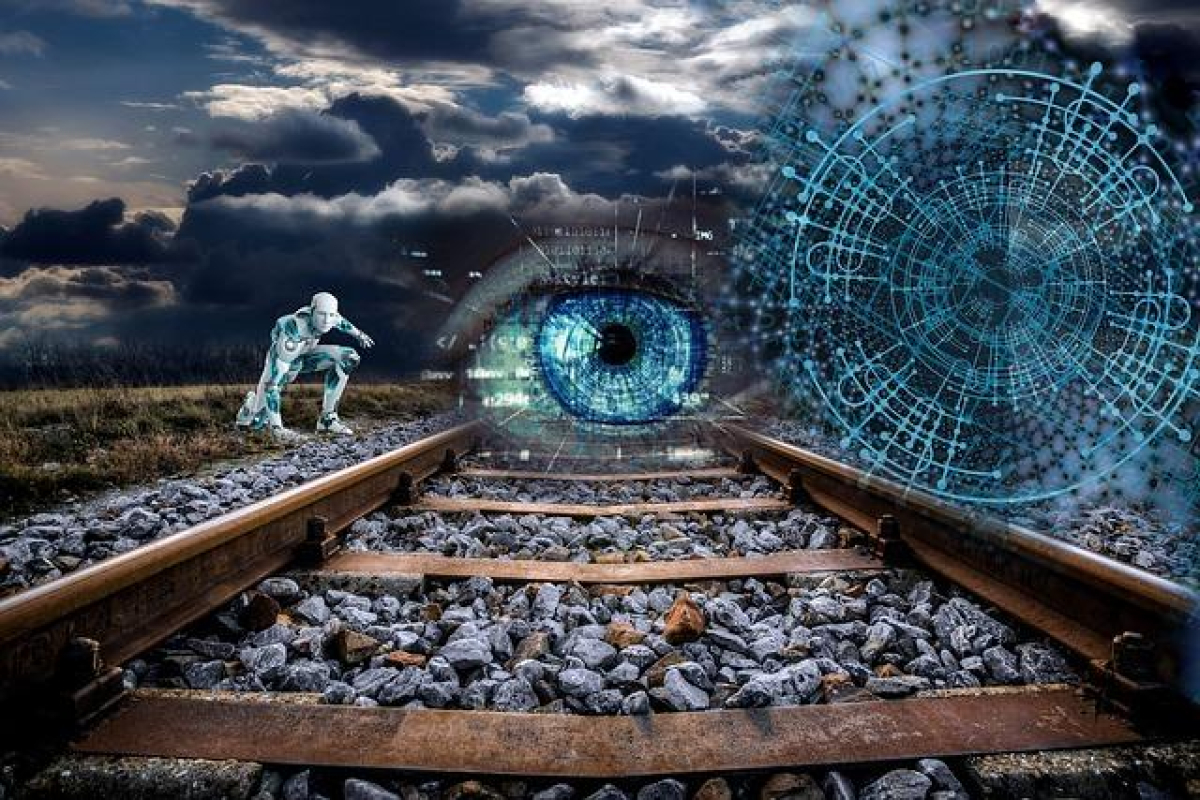
The contentious nature of generative AI
Generative AI’s training data origins spark controversy, as AI companies have not been transparent about their datasets, which often contain copyrighted material. Legal battles are determining whether this constitutes fair use. Additionally, there’s concern over the potential job displacement for creatives and various professionals due to generative AI’s capabilities. Moreover, the technology’s misuse for scams, misinformation, and other harmful activities poses significant risks, despite safeguards in place.
The dual potential of generative AI
Despite these concerns, many believe generative AI can enhance productivity and foster new creative avenues. As we navigate the potential pitfalls and breakthroughs, understanding these models becomes increasingly vital for those with a technological inclination. It’s up to us to manage these systems, improve future iterations, and harness their capabilities for the greater good.