Stable Diffusion 3 research findings
Discover the breakthroughs of Stable Diffusion 3 in our latest research paper. Unveiling superior text-to-image capabilities and innovative architecture, it's set to redefine AI artistry. Dive into the future of
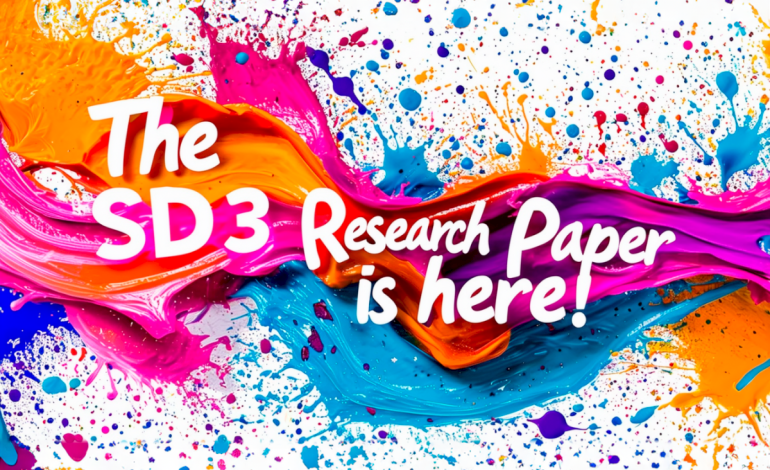
We are excited to share a comprehensive analysis of the latest advancements in AI-driven image generation, specifically focusing on the capabilities of Stable Diffusion 3. This groundbreaking research paper by Stability AI, soon to be available on arXiv, provides an in-depth look at the technology that sets this model apart from its predecessors and competitors.
Advancements in AI-driven image synthesis
The team behind Stable Diffusion 3 has made significant strides in the realm of text-to-image conversion, surpassing other leading systems such as DALL·E 3, Midjourney v6, and Ideogram v1. This is particularly evident in the model’s ability to adhere to textual prompts and its proficiency in rendering typography, as confirmed by evaluations based on human preferences.
Introducing the Multimodal Diffusion Transformer
At the core of these enhancements is the new Multimodal Diffusion Transformer (MMDiT) architecture. This innovative design employs distinct weight sets for processing image and language data, which has resulted in a marked improvement in text comprehension and spelling in comparison to earlier iterations of the model.
Comparative performance analysis
AI Media Cafe has conducted a thorough comparison of Stable Diffusion 3’s output with a variety of both open and closed-source models, including SDXL, SDXL Turbo, Stable Cascade, Playground v2.5, Pixart-α, DALL·E 3, Midjourney v6, and Ideogram v1. Human evaluators were tasked with assessing the outputs based on their adherence to the given prompts, the quality of text rendering, and overall visual aesthetics. The findings indicate that Stable Diffusion 3 either matches or exceeds the performance of current top-tier text-to-image generation systems across these metrics.
Optimization and accessibility
In preliminary tests on consumer-grade hardware, the most robust SD3 model, boasting 8 billion parameters, was able to fit within the 24GB VRAM of an RTX 4090 graphics card. It took approximately 34 seconds to generate a 1024×1024 resolution image using 50 sampling steps. To ensure broader accessibility, the initial release of Stable Diffusion 3 will include multiple model variations, ranging from 800 million to 8 billion parameters, thereby reducing hardware constraints for users.
Join the forefront of AI innovation
For those eager to experience the early preview of Stable Diffusion 3, AI Media Cafe invites you to join the waitlist. This opportunity will allow you to be among the first to explore the potential of this state-of-the-art model and contribute to the evolution of AI media technology.
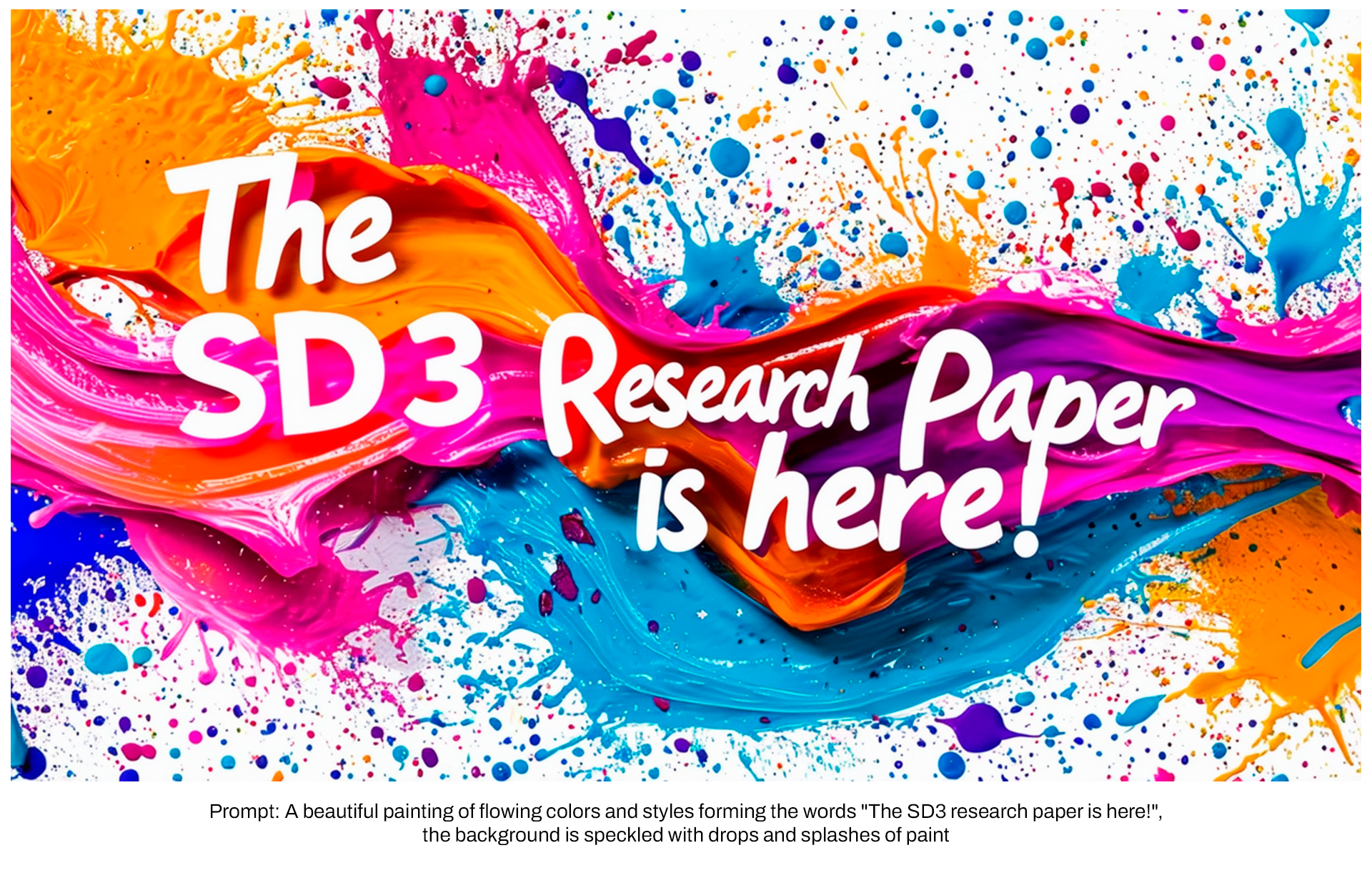
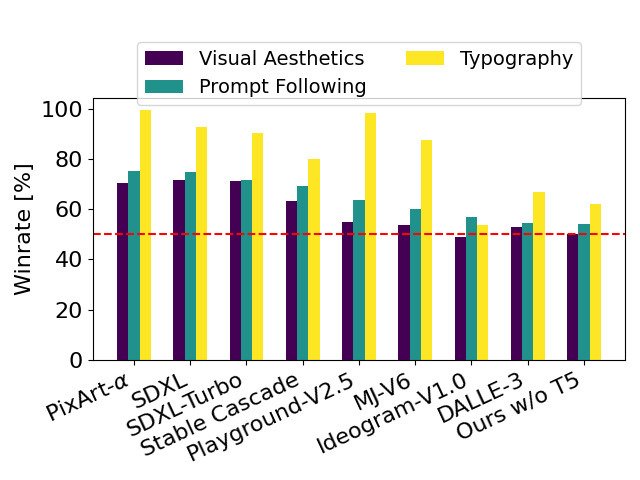
Exploring the intricacies of multimodal AI architecture
At the heart of the latest advancements in AI-generated imagery lies a sophisticated architecture known as MMDiT, which stands for Modified Multimodal Diffusion Transformer. This innovative framework is adept at interpreting and integrating different types of data inputs, namely textual descriptions and visual content. The AI Media Cafe delves into how MMDiT leverages a trio of text embedders—two from the CLIP models and one from T5—to effectively encode textual nuances, while an enhanced autoencoding model adeptly handles the visual aspects, transforming image tokens into a comprehensible format.
Unifying text and image embeddings
The SD3 architecture, which is an evolution of the Diffusion Transformer (DiT) conceptualized by Peebles & Xie in 2023, introduces a novel approach to processing distinct data modalities. It employs separate weight sets for text and image embeddings, allowing each to maintain its unique properties. However, as depicted in the accompanying visual representation, these two modalities are not entirely isolated. They are intricately linked during the attention phase, enabling a seamless exchange of information that enhances the model’s ability to generate coherent and typographically sound outputs.
Extending to new horizons
One of the most compelling aspects of this architecture is its scalability. The design facilitates the incorporation of additional modalities, such as video content, which is further elaborated in our research publication. This flexibility paves the way for future enhancements and broader applications of the technology.
Enhanced prompt adherence in image generation
Stable Diffusion 3 has made significant strides in its ability to adhere to prompts, a feature that allows for the creation of images with a keen focus on diverse themes and subjects. This capability is a testament to the model’s refined understanding of user input and its translation into visually stunning and relevant imagery.
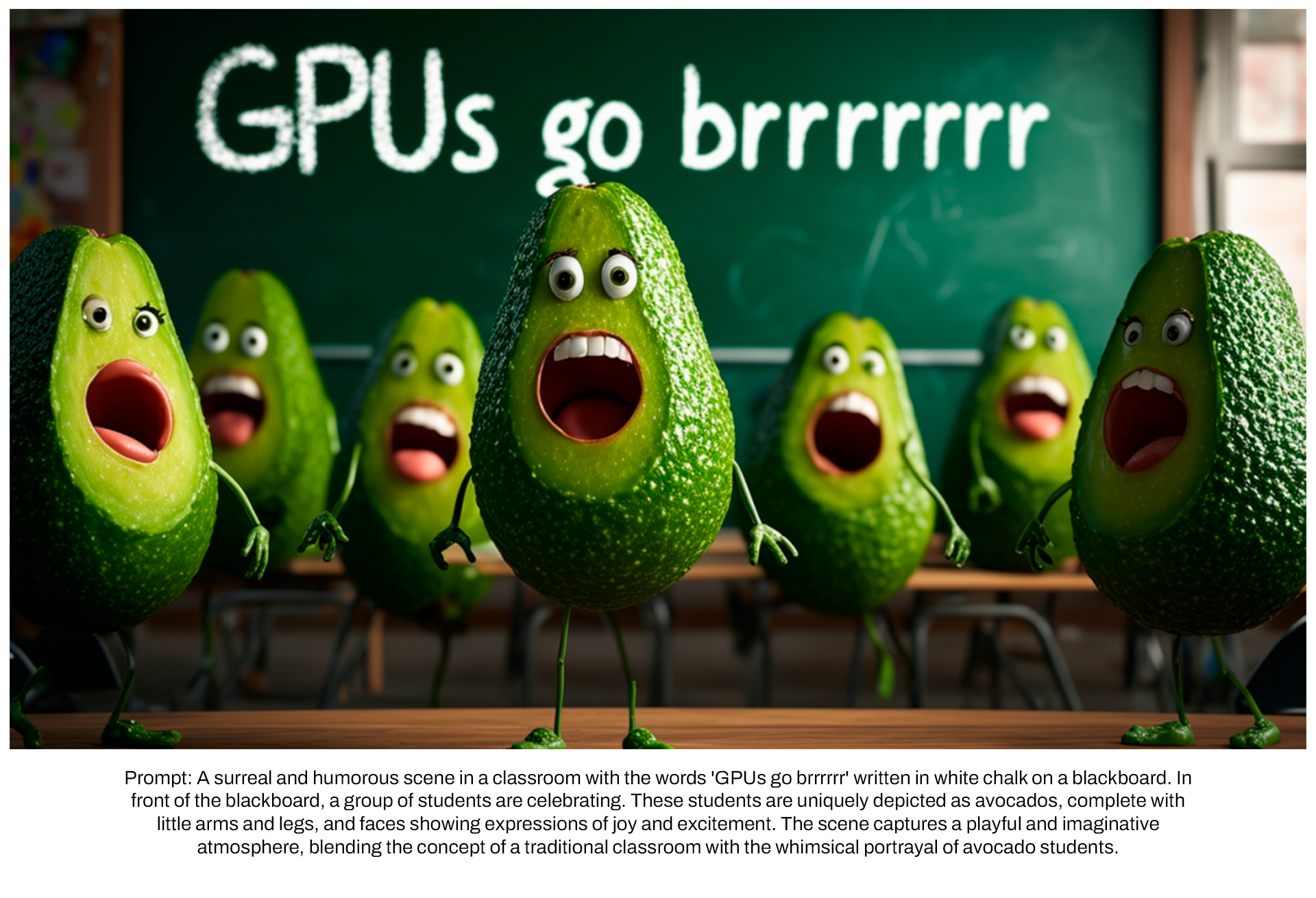
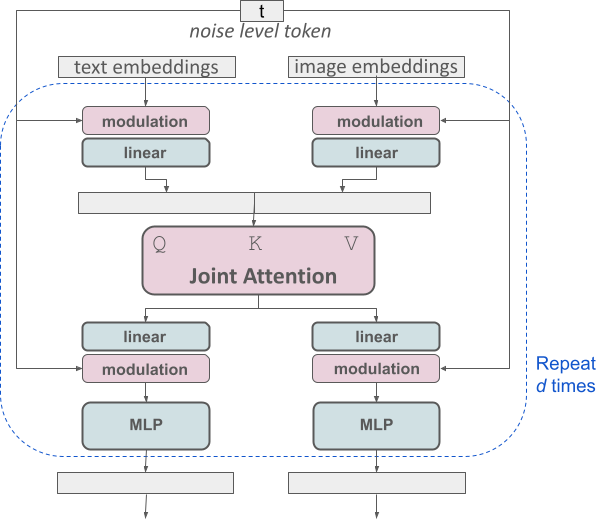
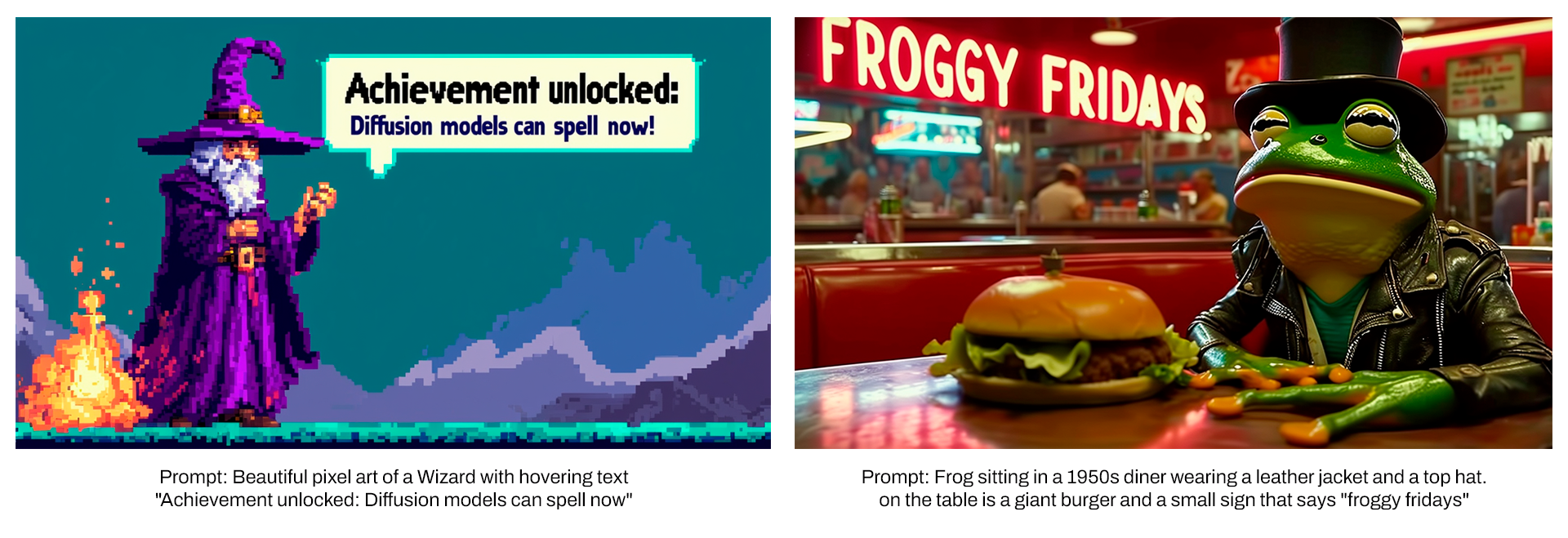
Enhancing diffusion models through strategic weighting
At AI Media Cafe, we delve into the latest advancements in AI-generated imagery, particularly focusing on the innovative Stable Diffusion 3. This model incorporates a technique known as Rectified Flow (RF), referenced by researchers such as Liu et al. (2022), Albergo & Vanden-Eijnden (2022), and Lipman et al. (2023). RF connects data and noise linearly during the training phase, paving the way for more direct inference routes and enabling image sampling in fewer steps. A groundbreaking addition to this process is a new sampling schedule that emphasizes the central segments of these trajectories. This emphasis is based on the premise that these segments pose more complex prediction challenges. Our comparative analysis, which included 60 different diffusion trajectories like LDM, EDM, and ADM, spanned various datasets, metrics, and sampler configurations. The findings reveal that while traditional RF models excel in limited-step sampling, their advantage diminishes as the number of steps increases. However, our modified RF approach with reweighted trajectories consistently enhances performance across the board.
Expanding the capabilities of transformer models
Our team conducted an extensive scaling study on text-to-image synthesis using the reweighted RF method combined with the MMDiT architecture. We trained a spectrum of models, from those with 450 million parameters across 15 blocks to behemoths with 8 billion parameters spread over 38 blocks. The validation loss showed a steady decline, correlating with increases in both model size and training duration. To ascertain if this loss reduction translated to tangible output enhancements, we evaluated the models using automatic image-alignment metrics like GenEval and human preference scores (ELO). The results confirmed a robust link between these metrics and the validation loss, suggesting the latter is a reliable indicator of model quality. Moreover, the absence of a saturation point in the scaling trend suggests that there is still room for further improvements in our models’ performance.
Adaptable text encoding strategies
The memory demands of Stable Diffusion 3 can be significantly reduced by omitting the 4.7 billion parameter T5 text encoder during inference, with only a marginal impact on performance. This adjustment slightly diminishes text adherence, as evidenced by a win rate drop from 50% to 46%, yet it does not compromise the visual appeal of the generated images. Nonetheless, for those seeking to leverage the full capabilities of SD3 in text generation, the inclusion of the T5 encoder is advisable. Without it, we’ve observed a more pronounced decline in the quality of typography generation, with the win rate falling to 38%. These findings underscore the importance of the T5 encoder in maintaining the integrity of text-based outputs.
Exploring the intricacies of MMDiT and Rectified Flows
At AI Media Cafe, we delve into the latest advancements in artificial intelligence, particularly those that shape the media landscape. A recent study has shed light on the impact of removing T5, a text-to-text transfer transformer, during the inference phase. This action has been observed to cause substantial declines in the quality of outputs, especially when dealing with complex prompts that include a multitude of details or extensive written content. The accompanying visual representation illustrates the stark contrast in results with three varied samples for each scenario.
Deep dive into Stable Diffusion 3’s research
For enthusiasts eager to gain a deeper understanding of the mechanisms behind Stable Diffusion 3, including MMDiT and Rectified Flows, the full research paper offers a wealth of information. It provides a comprehensive analysis of the technology and the principles that underpin these innovative approaches to AI-driven media generation.
Join the conversation and stay informed
Keeping abreast of the rapid developments in AI technology is crucial for those with a vested interest in the field. AI Media Cafe encourages readers to follow our updates across various social platforms. By connecting with us on Twitter, Instagram, LinkedIn, and participating in our Discord Community, you can stay informed about the latest news, engage with fellow tech enthusiasts, and contribute to discussions that shape the future of AI in media.