Revolutionizing AI image generation with one-step diffusion
Revolutionize your visuals with MIT's breakthrough AI! Discover how a one-step generator creates stunning images in a fraction of the time. Dive into the future of image generation now!
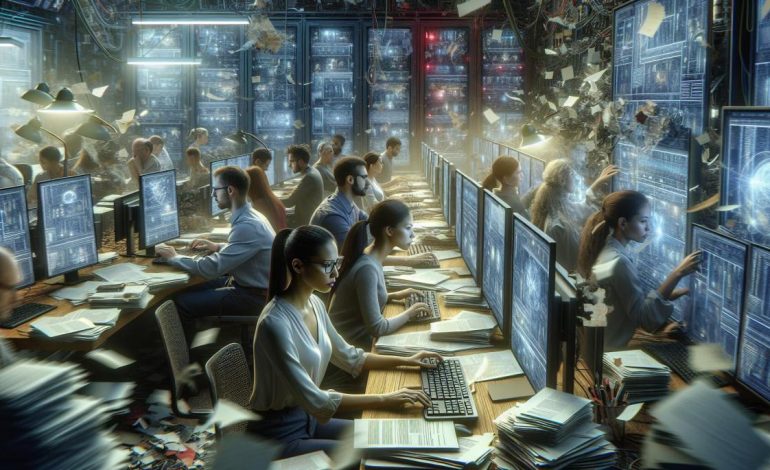
Researchers at the Massachusetts Institute of Technology (MIT) have made a significant breakthrough in the field of artificial intelligence by developing a one-step AI image generator. This innovative approach condenses the complex multi-stage process typical of traditional diffusion models into a singular, streamlined action.
By employing a novel training technique, the team has managed to accelerate image generation by a factor of 30. This leap in efficiency is achieved through a teacher-student dynamic, where a newly designed computer model learns to replicate the intricate procedures of its predecessors, which are known for their high-quality image outputs. The process, termed distribution matching distillation (DMD), not only speeds up the creation of images but also ensures that the quality remains uncompromised.
Enhancing diffusion models for rapid visual content creation
The DMD technique represents a significant advancement in optimizing well-known diffusion models such as Stable Diffusion and DALLE-3. It offers a 30-fold improvement in processing speed while maintaining, and in some cases enhancing, the visual quality of the output. This method marries the concepts of diffusion models and generative adversarial networks (GANs), enabling the generation of visual content in a single step rather than the hundred iterative stages previously required. The potential of DMD lies in its ability to become a groundbreaking generative modeling technique that doesn’t sacrifice speed for quality.
At the core of DMD are two essential components:
- Regression loss: This element anchors the image space mapping, providing a stable foundation for training.
- Distribution matching loss: It ensures that the likelihood of the student model generating a particular image aligns with the actual frequency of that image’s occurrence in the real world.
The DMD framework utilizes a pair of diffusion models as benchmarks to differentiate between synthetic and authentic images, which in turn guides the training of the rapid one-step generator. The system’s increased speed is a result of training a new network to minimize the divergence between its generated images and the dataset used by conventional diffusion models.
Tianwei Yin, an MIT PhD candidate and the lead researcher on the DMD project, explains that the breakthrough comes from approximating gradients that refine the new model using two diffusion models. This strategy allows the team to distill the knowledge from the original, more intricate model into a simpler, faster one, effectively sidestepping the instability and mode collapse issues often associated with GANs.
The team leveraged pre-trained networks to streamline the process for the new student model. By replicating and fine-tuning parameters from the original models, they achieved rapid training convergence for their new model, which is capable of producing high-quality images on the same architectural foundation.
When put to the test, the DMD model demonstrated consistent performance against traditional methods. It is the first one-step diffusion technique to produce images that rival those from the original, more complex models on the popular ImageNet benchmark for generating images based on specific classes. The model’s Fréchet inception distance (FID) score of just 0.3 is particularly impressive, as FID is a measure of the quality and diversity of generated images.
Applications and future potential of DMD
DMD excels in various applications, including industrial-scale text-to-image generation and achieving state-of-the-art performance in one-step generation. The effectiveness of the images produced by DMD is intrinsically linked to the capabilities of the teaching model used during the distillation process. With Stable Diffusion v1.5 as the current teacher model, the student model inherits certain limitations, such as the ability to draw small faces and detailed text representations. This suggests that employing more advanced teacher models could further enhance the quality of DMD-generated images.
Fredo Durand, an MIT professor and principal investigator at CSAIL, emphasizes the significance of reducing the number of iterations in diffusion models, a goal that has been pursued since their inception. The advent of single-step image generation is a game-changer, promising to slash compute costs and expedite the creative process.
Alexei Efros, a professor at the University of California at Berkeley who was not part of the study, commends the successful fusion of diffusion models’ versatility and visual quality with the real-time performance of GANs. He anticipates that this development will unlock new possibilities for high-quality, real-time visual editing.
Journal Reference:
- Tianwei Yin, Michaël Gharbi, Richard Zhang, Eli Shechtman, Fredo Durand, William T. Freeman, Taesung Park. One-step Diffusion with Distribution Matching Distillation. DOI: 10.48550/arXiv.2311.18828